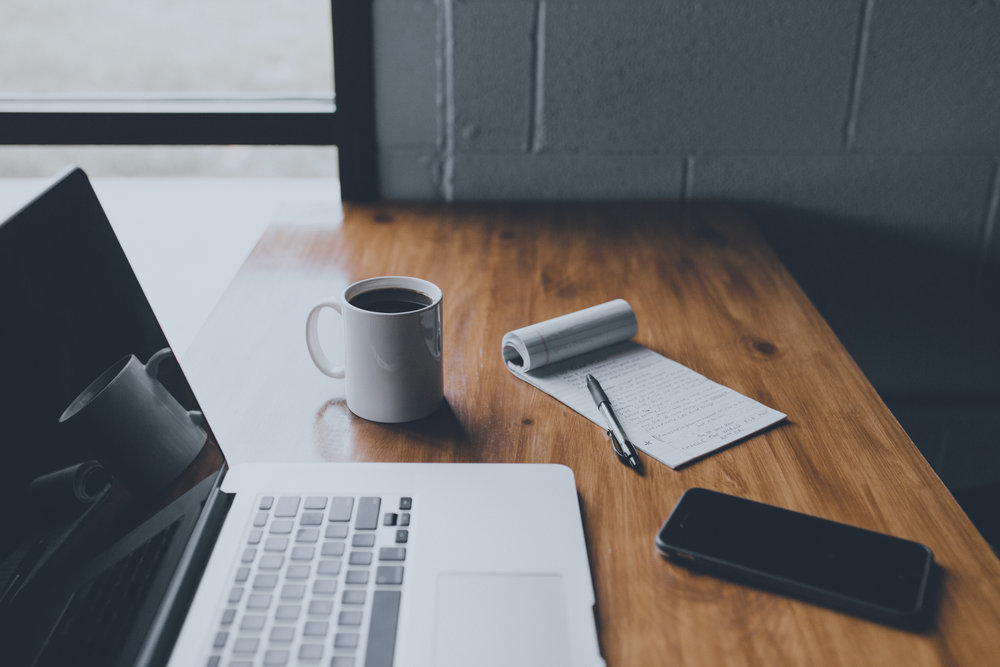
WHY WE’RE DIFFERENT
On December 3, 2018, FinCEN and Federal Regulators issued a joint statement, recommending financial institutions to use Artificial Intelligence and Machine Learning for AML compliance. Therefore, it is the time for you to upgrade to Patriot Officer®, which uses the most advanced Artificial Intelligence and Machine Learning technologies and has been awarded over ten (10) patents.
In the field of Artificial Intelligence, a “negative” is a set of data that has not been triggered as an alert. A “true negative” is a set of data that has not been triggered as an alert, and does not constitute a true case. A “false negative” is a set of data that has not been triggered as an alert; however, it comprises a true case that the system has missed. Similarly, a “positive” is a set of data that has been triggered as an alert. A “true positive” is an alert that turns out to be a true case. A “false positive” is an alert that does not turn out to be a true case.
A “false negative” money laundering case may cause a financial institution to be penalized by the U.S. government if the “false negative” case (i.e., a true money laundering case) is discovered by the U.S. government later. Therefore, the minimum standard for BSA/AML compliance is “no false negatives.”
Three different types of BSA/AML systems are analyzed below:
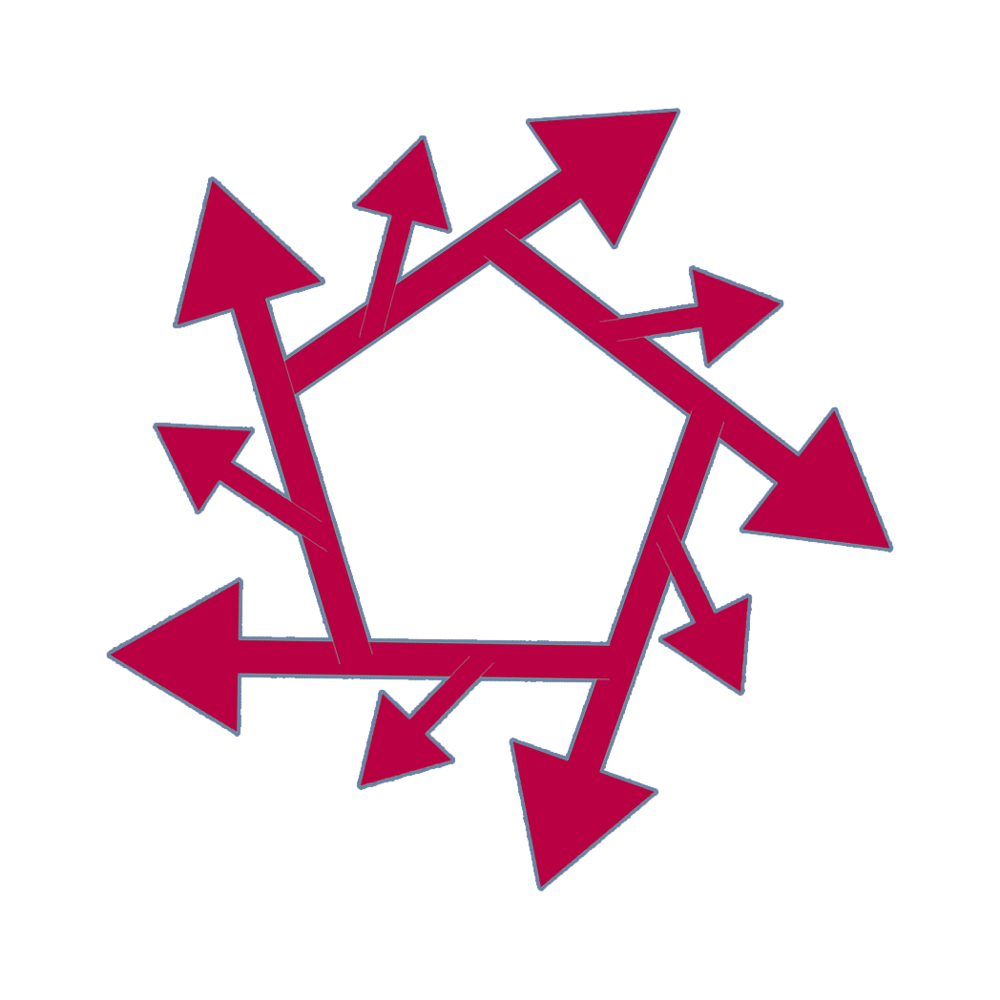
Rule-Based Systems
Many financial institutions have used the rule-based approach which can trigger many alerts. For example, there are over 200 countries in the world. If a financial institution wants to monitor the wire transfers to (or from) each country, the financial institution may have “200+ branches at the country decision node of the decision tree” if a rule-based approach is used. As another example, there are thousands of industries. If a financial institution wants to monitor the wire transfers to (or from) each industry, the financial institution may have “thousands of branches at the industry decision node of the decision tree” if a rule-based approach is used. In fact, “country” and “industry” are just two of many risk categories that have money laundering exposure. Similarly, “wire transfer” is just one of many types of transactions that carries money laundering risk. For example, cash, check, ACH, ATM, credit card, debit card, letter of credit, etc. are other types of transactions.
There are various money laundering risk factors. If we use the terminology in Machine Learning to describe this challenge, there are millions of combinations of branches to form a path from the root of a decision tree to the leaf nodes of the decision tree. In other words, millions of rules are required to cover the entire scope of money laundering risk if a rule-based system is used to detect suspicious money laundering activities. A rule-based system with less than millions of rules may have many “false negatives” (i.e., the system has missed true money laundering cases and cannot meet the minimum standard of BSA/AML compliance) and many “false positives” (i.e., the leaf nodes of the decision tree have very high impurity and cannot truly achieve the goal of classification). This is the reason why a financial institution needs to hire many BSA/AML experts to review a large number of alerts if a rule-based approach is used.
The reality is that rule-based systems cannot comply with the minimum BSA/AML compliance standard of “no false negatives” because financial institutions cannot afford to use a rule-based system that has millions of rules.
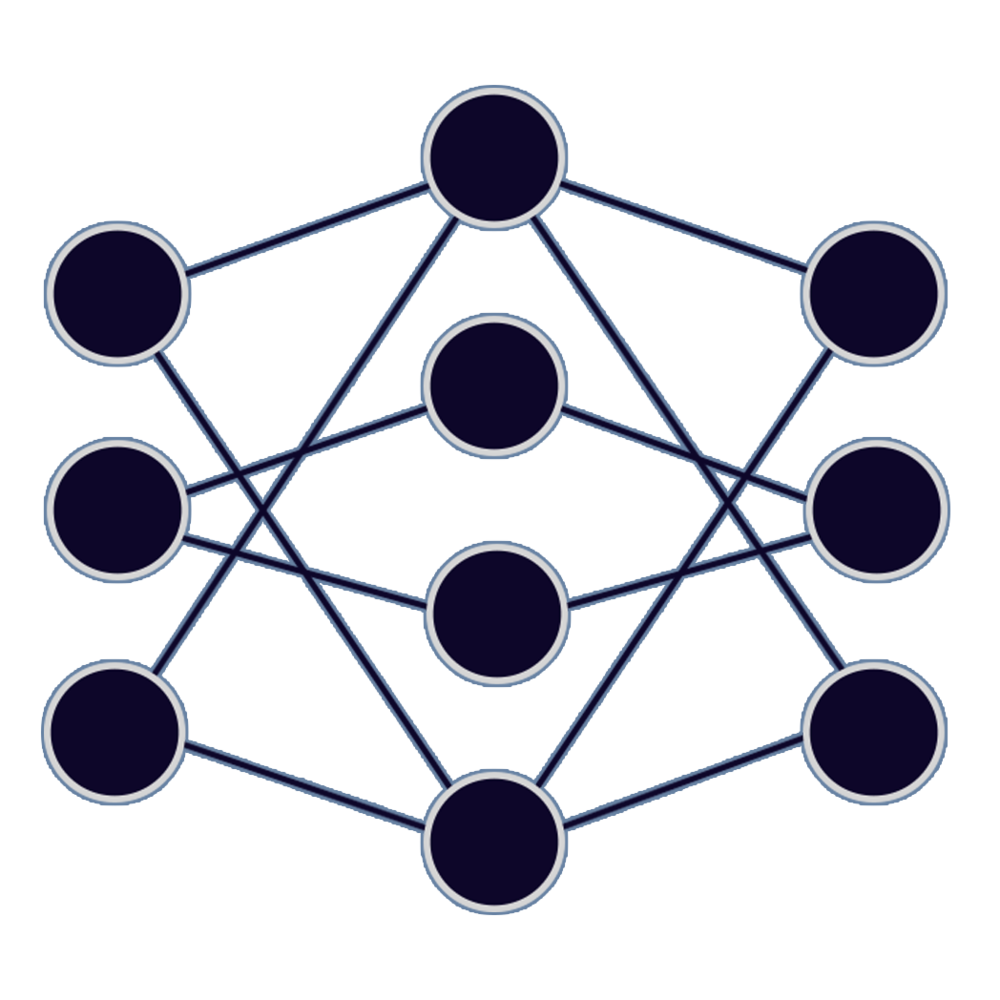
Behavior-Based Systems
Some vendors promote that “behavior-based” systems produce fewer alerts than rule-based systems. This is a false promotion. Behavior-based systems use “changes of behavior” to trigger alerts. A behavior change may happen in a fraud case committed by a third party (e.g., stolen credit card, counterfeit check, etc.) because the victim and the fraudster are two different persons and it is very likely that they behave differently.
In reality, behavior-based systems have missed many true money laundering cases (i.e., behavior-based systems have a large number of false negatives) because money laundering can be conducted without any behavior change. For example, a human trafficker can routinely send funds to a remote accomplice without changing behavior. There are countless money laundering activities that can be conducted without any behavior changes. This is why behavior-based systems have failed regulatory examinations.
The truth is that “behavior changes” only cover a small number of the branches of the decision tree that detects money laundering activities. Because behavior-based systems only cover a small portion of the money laundering risk, they trigger fewer alerts. At the same time, because behavior-based systems only cover a small portion of the money laundering risk, they produce many false negatives (i.e., they miss true money laundering cases).
Moreover, behavior-based AML systems often falsely detect fraud cases as money laundering cases. This occurs because behavior changes often occur after the fraudsters have stolen the checks, credit cards, etc. from the victims. This is the reason why vendors of behavior-based systems must claim that their systems can detect money laundering cases and fraud cases together. The truth is that because behavior-based systems cannot distinguish between money laundering cases and fraud cases, these systems have no choice but to mix fraud cases together with money laundering cases.
For fraud cases committed by third parties, the persons involved in the detected cases are actually victims of fraud, not money launderers. In essence, behavior-based systems falsely detect victims of fraud (i.e., innocent customers) as money launderers (i.e., criminals). The fact is that behavior-based systems not only have many false negatives, but also have many false positives when they are used for AML monitoring purposes. It is predicted that behavior-based systems may disappear soon in the BSA/AML industry because government regulators and examiners have become more knowledgeable about the differences between money laundering and fraud. Many intelligent BSA/AML experts have already decided that they do not want to associate with behavior-based systems.
When BSA/AML experts examine the detection algorithms of a behavior-based system, they can easily uncover the flaws in the behavior-based system. To hide their flaws, behavior-based systems are usually designed like a black box so that no one can find out how alerts are actually triggered by the systems. As a result, the users of behavior-based systems do not truly know the reasons behind each alert. This is another reason why the behavior-based system failed regulatory examinations.
Furthermore, behavior-based systems do not create a true risk score for each customer. Instead, behavior-based systems only create behavior scores for those customers who have changes of behavior. Therefore, to comply with the BSA/AML Examination Manual, financial institutions that use behavior-based systems need to use spreadsheets to calculate the risk score of each customer in order to identify the higher-risk customers. This is a labor-intensive and time-consuming task for which financial institutions do not have time. These systems cannot identify all the higher-risk customers as mandated by the BSA/AML Examination Manual.
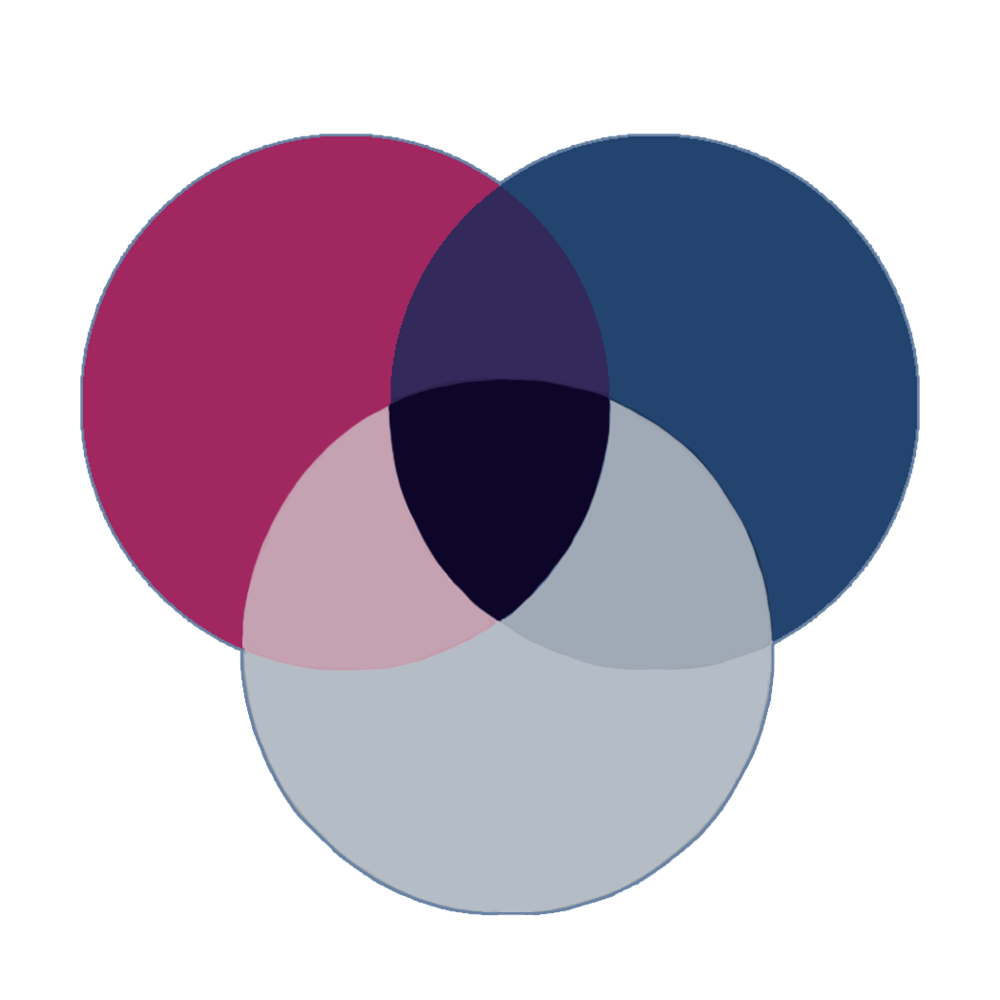
Risk-Based Systems
Thanks to the intelligence of the Federal Financial Institutions Examination Council (“FFIEC”), the BSA/AML Examination Manual was revised in April 2010 to provide an official solution. In this revised manual, the FFIEC replaced the phrase “high-risk customer” with “higher-risk customer” throughout the manual. On page 57 of the BSA/AML Examination Manual published in February 2015, the title of the section is “Enhanced Due Diligence for Higher-Risk Customers.” The manual further states that “…Higher-risk customers and their transactions should be reviewed more closely at account opening and more frequently throughout the term of their relationship with the bank.”
In order to fully comply with the BSA/AML Examination Manual, a financial institution must (1) identify the higher-risk customers, (2) conduct EDD on these higher-risk customers, and (3) monitor these higher-risk customers and their transactions more closely as long as their accounts are open.
By conducting Risk Scoring (U.S. Patent) at account opening and on an ongoing basis throughout the term of a customer’s relationship with the financial institution, Patriot Officer® can automatically and dynamically calculate a risk score for each customer. Findings are based on millions of combinations of risk factors, including products, services, customers, entities, transactions, and geographic locations, etc. as required by the BSA/AML Examination Manual.
Once a risk score of a customer is obtained through the Risk Scoring process, a financial institution can easily identify the customer as having a “higher risk” if the risk score is higher than a certain value determined by the policy of the financial institution and can conduct EDD on the customer accordingly.
The effect of a “risk score” is very similar to the effect of a “credit score.” Thirty years ago, a financial institution might have spent a week to investigate a person’s background before the financial institution issued a car loan to this person. Today, a decision to issue a car loan can be made within a few minutes based on the credit score of the loan applicant.
Similarly, a BSA/AML expert can use the risk scores to quickly identify the higher-risk customers without investigating potential risk factors associated with each customer.
Because Patriot Officer® has included all money laundering risk factors of a customer into the Risk-Scoring process, Patriot Officer® has equivalently consolidated the effects of millions of rules into one risk score, and effectively eliminates the need of using millions of rules. More importantly, by eliminating millions of rules, Patriot Officer® has eliminated all redundant alerts associated with those millions of rules.
Patriot Officer® is the only BSA/AML solution that covers all money laundering risk factors while all rule-based systems and behavior-based systems in the marketplace can only cover a very small number of money laundering risk factors.
CONCLUSION
Neither “rule-based” systems nor “behavior-based” systems can meet the minimum BSA/AML compliance standard of “no false negatives.” Furthermore, neither “rule-based” systems nor “behavior-based” systems can comply with the “risk-based” requirements mandated by the BSA/AML Examination Manual. Only the risk-based solution provided by Patriot Officer® has fully complied with the BSA/AML Examination Manual published by the FFIEC.
Patriot Officer® uses Machine Learning technology, which is the most advanced technology in the field of Artificial Intelligence, to produce the most comprehensive Risk Model, which eliminates the need to use millions of rules. Patriot Officer® has “No False Negatives and minimum false positives.”
The Risk Model of Patriot Officer® has been implemented in the field for over ten (10) years and has been proven to be the most powerful solution to BSA/AML compliance. Furthermore, the Patriot Officer® system is designed to be transparent. Numerous examiners and auditors have already examined the Risk Model of Patriot Officer®. The Risk Model and detection scenarios are user-configurable, viewable, verifiable, and auditable.
Patriot Officer® has incorporated over 10 patents to provide users with the most advanced A.I. – powered technologies, including Risk Scoring, Multidimensional Risk-Based Detection, Multidimensional Risk-Directed Detection, Multidimensional Risk-Based Data Mining, and Multidimensional Risk-Based Peer Group Analysis. These Risk-Based technologies are the next-generation technologies which will empower financial institutions to comply with the Risk-Based requirements mandated by the BSA/AML Examination Manual published by the FFIEC.
Not only has Patriot Officer® complied with the BSA/AML Examination Manual, Patriot Officer® has also complied with the BSA/AML standard of “no false negatives.” By contrast, other BSA/AML systems produce many false negatives (i.e., missing true money laundering cases). Because of the patent protection, no other vendor can copy the design of Patriot Officer®. Therefore, in due time, all legacy BSA/AML systems will fail regulatory examinations.
In addition to Patriot Officer®, AI OASIS® has produced the world’s first and only financial crimes alarm system, Enquirer Officer®, which empowers a financial institution to discover hidden information about its customers, non-customers, and beneficial owners; receive early warnings about them; and block criminals from opening accounts with the financial institution. Furthermore, we have produced the most advanced and comprehensive fraud prevention system, Guardian Officer®, which empowers a financial institution to detect all types of fraud in advance and protects the financial institution against losses and damages. In addition, we have produced the most innovative consumer protection system, Champion Officer®, which protects consumers against identity theft and financial crimes.
Patriot Officer®, Guardian Officer®, Enquirer Officer® and Champion Officer® have jointly established the most powerful Global Shield to protect financial institutions and consumers against all types of financial crimes, losses, and damages. Contact AI OASIS® today to ensure your future success in BSA/AML compliance, Risk Management, Fraud Elimination, Financial Crimes Prevention, and Consumer Protection. AI OASIS® is the destination where your safe and happy life starts.
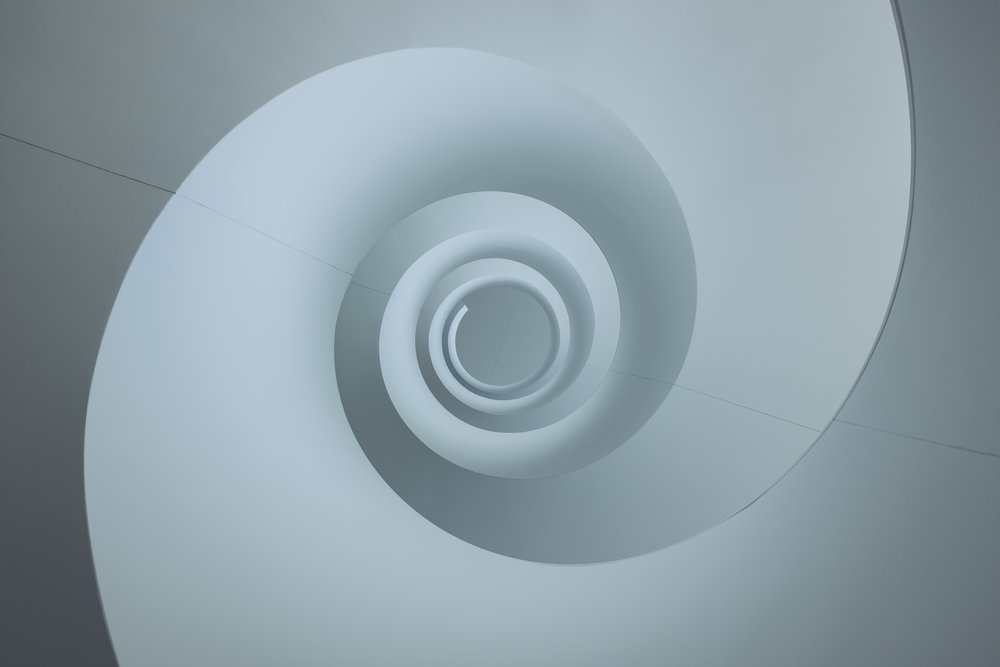
WE’VE DONE THE
RESEARCH
The following white papers have been read by millions of professionals and experts in the financial industry worldwide. We would highly recommend the visitors to this AI OASIS® website to download these white papers. Please distribute to friends and colleagues as you see fit.
No False Positives and No False Negatives
The Ultimate Solution to Preventing Fraud
Illicit Proceeds Tracking System
The Only Money-Making AML Solution
No False Negatives
The Minimum Standard for BSA/AML Compliance
AML Integrity
The Foundation of Our Homeland Security
Rejected By Safe Harbor
The Beginning of Endless Lawsuits and Regulatory Penalties